Recently I walked the first half of the Cotswold Way (from North to South). While planning the second half, working out how many miles to attempt each day, I wanted to compare the terrain for the two halves of the walk.
The Ordnance Survey provide, as Open Data, a Digital Terrain Model (DTM) based on a 50m grid size, which is a good enough resolution for my purposes. Unfortunately the 158Mb download is for the whole of Great Britain. Another added complication is that the zip file contains a number of other zip files, one for each 10km tile. I wanted to automate the process of extracting only the necessary tiles and adding them as layers in QGIS.
The first stage was to get the 10km grid as a QGIS layer, to determine which tiles I needed. It is possible to download all of the OS grids as a geopackage. Here the 10km grid is shown along with the route of the Cotswold Way (downloaded as a gpx file from the National trails website). I have added a 1km buffer to the route since it will be useful to also get tiles very close, but not intersecting the route (e.g. tile ST89):
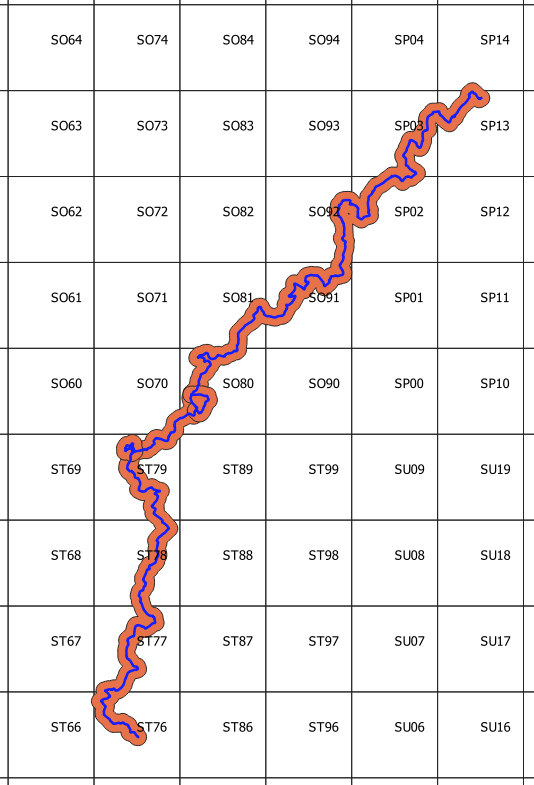